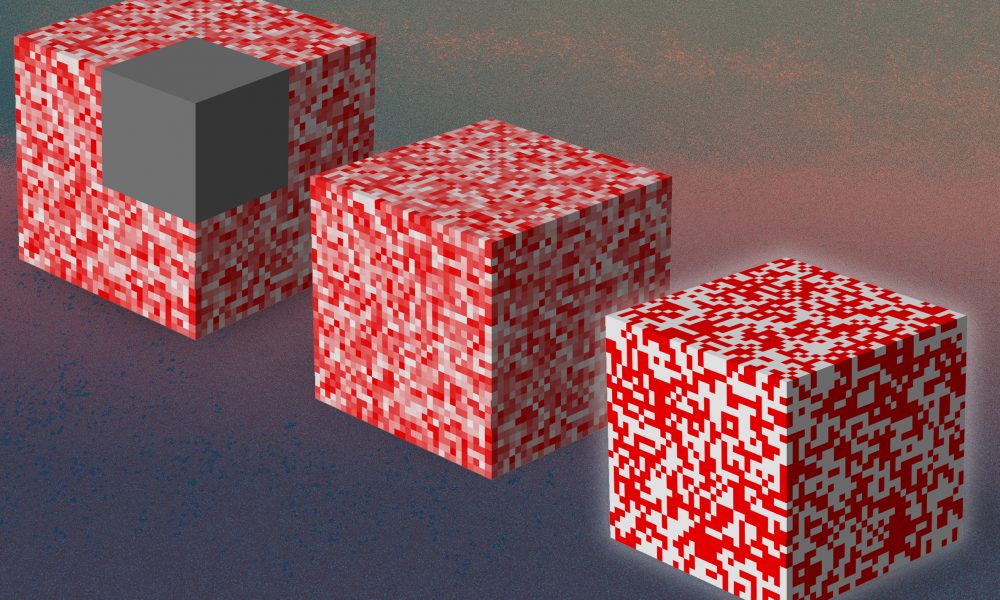
This Deep Learning Tool Enables Engineers to Look “Inside” Objects!
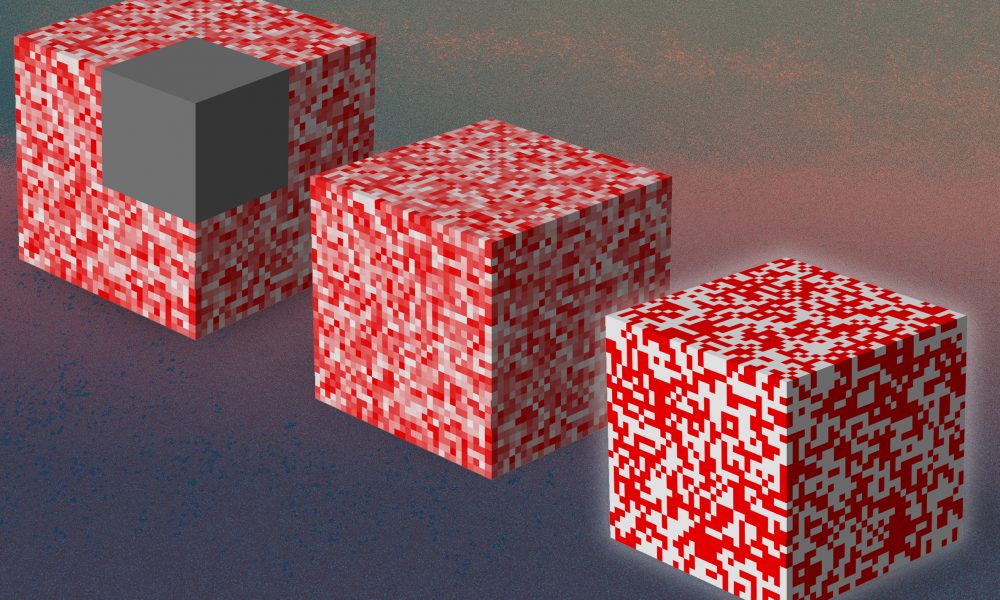
The days of engineers painstakingly examining the interior of materials are over. Thanks to artificial intelligence (AI) and deep learning, engineers can quickly analyze the properties of a material’s surface and determine what is happening beneath it. This deep learning method provides detailed information about internal structures, voids, and cracks – all from exterior data points.
Let’s take a closer look at this deep learning method that is revolutionizing the material industry.
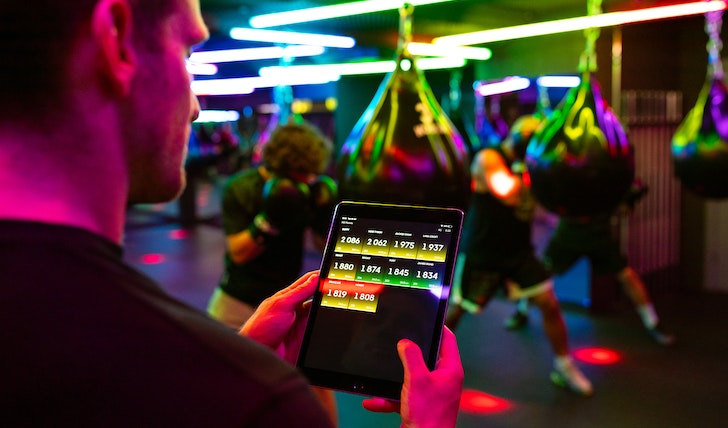
This Engineer / Pexels | According to the early updates, this deep learning method can be applied to all particles – from the parts of airplanes to cars.
How Does Deep Learning Work?
Deep learning uses large datasets to train algorithms to recognize patterns and spot correlations between input features and output variables. In this case, the input features would be exterior conditions. These include:
- Temperature
- Pressure
- Color
Anything observable from the outside of a material. The output variable could be any internal phenomenon or structure that needs to be identified within the material.
The algorithms learn from the datasets to accurately identify internal phenomena and provide useful information to engineers. This is done by using neural networks.
Essentially, these are computer systems modeled after biological neurons in the brain that form connections between input features and output variables. With enough data, these neural networks can accurately predict what is happening inside a material based on exterior conditions.
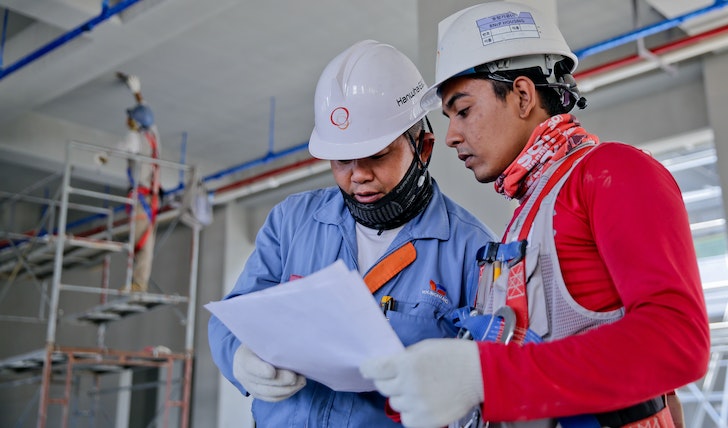
Anamul / Pexels | This Deep Learning method analyzes the interior of materials right from the outside.
Advantages of Deep Learning
Deep learning has many advantages over traditional engineering methods for analyzing materials. It offers improved accuracy and efficiency while reducing the time needed to gain accurate results from materials testing and eliminating potential human errors during manual analysis.
Additionally, deep learning eliminates certain limitations of traditional measurement techniques, such as limited sample size or access to hard-to-reach locations.
The Future of Deep Learning in Material Analysis
As deep learning technology continues to develop, engineers will be able to use it for a variety of materials analysis applications. This includes analyzing the internal structure of components such as metals, plastics, and composites used in medical devices and automotive parts. The technology can also be utilized by structural engineers who examine bridges and buildings for structural defect detection.
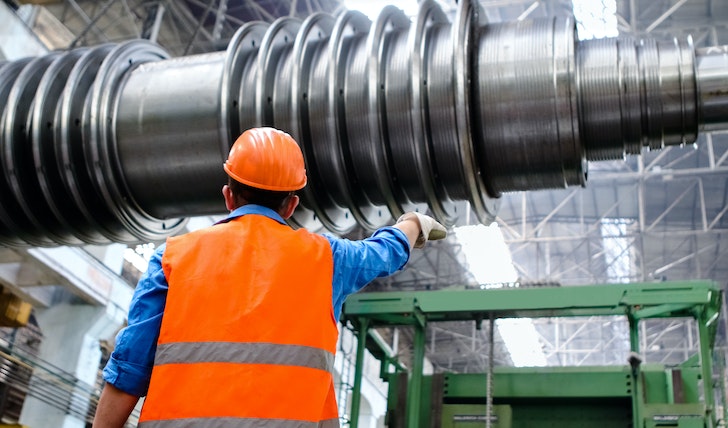
Katerina / Pexels | With the advancement of this Deep Learning method, engineers do not have to dig deep to figure out the malfunctioning in the interior of any material.
Deep learning has made an incredible impact on material analysis and engineering in general. With its ability to quickly analyze data from exterior conditions, engineers can now get detailed information about internal structures much faster than before. This new approach provides accurate results with improved efficiency, eliminating manual errors and saving time for engineers worldwide.
Parting Thoughts
Deep learning has revolutionized how engineers analyze materials, making the traditionally tedious task of material analysis much easier and faster. By using algorithms trained on large datasets, engineers can get detailed information about internal structures, voids, and cracks simply by observing the properties of the material’s surface.
This new approach provides accurate results with improved efficiency while eliminating manual errors and saving time for engineers around the globe. With continued development, deep learning will continue to have an incredible impact on material analysis and engineering as a whole.
More in Celebrity
-
`
Family Wellness Journeys: Where Health and Adventure Unite
In the hustle and bustle of parenting, where chaos often reigns supreme, there’s a rising trend that’s changing the game for...
December 14, 2023 -
`
Too Much Intake of Protein Is NOT As Healthy As You Might Think
Proteins are often hailed as the macronutrient monarchs of our diet, lauded for their muscle-mending and appetite-appeasing powers. But is there...
December 9, 2023 -
`
Popular Celebs Who Left Hollywood to Become Politicians
When we think of celebrities, we often picture the glitz and glamor of Hollywood, red carpets, and blockbuster premieres. However, some...
November 29, 2023 -
`
Marie Claire’s Favorite Sustainable Fashion Brands
Zedify Let’s start our countdown with a brand that is changing the game from the ground up: Zedify. While not your...
November 26, 2023 -
`
Striving or Thriving? Steps to Help Kids Find Balance and Purpose
In today’s fast-paced and achievement-oriented world, the pressure on children to excel academically, socially, and in extracurricular activities can be overwhelming....
November 19, 2023 -
`
How Sobriety Can Make You a Better Human Being
Sobriety is more than just abstaining from substances. It is a profound shift in perspective and lifestyle. This article will explore...
November 12, 2023 -
`
Why Hollywood Will Not Cast Shia LaBeouf Anymore
Remember the sweet-faced kid from “Even Stevens?” That bright-eyed boy who captivated our hearts in “Transformers?” Well, that Shia LaBeouf has...
November 1, 2023 -
`
The Nautilus Superyacht Submarine: A Subaquatic Oasis of Luxury
Imagine a world where you can trade the concrete jungle for the mysteries of the ocean depths while still enjoying the...
October 29, 2023 -
`
Peloton Recalls 2.2 Million Bikes Due to Safety Concerns: Here’s What You Need to Know
Peloton, a leading brand in the fitness industry, has issued a recall for their original Peloton bikes due to safety concerns....
October 28, 2023
You must be logged in to post a comment Login